Luhuan Wu
New York, NY
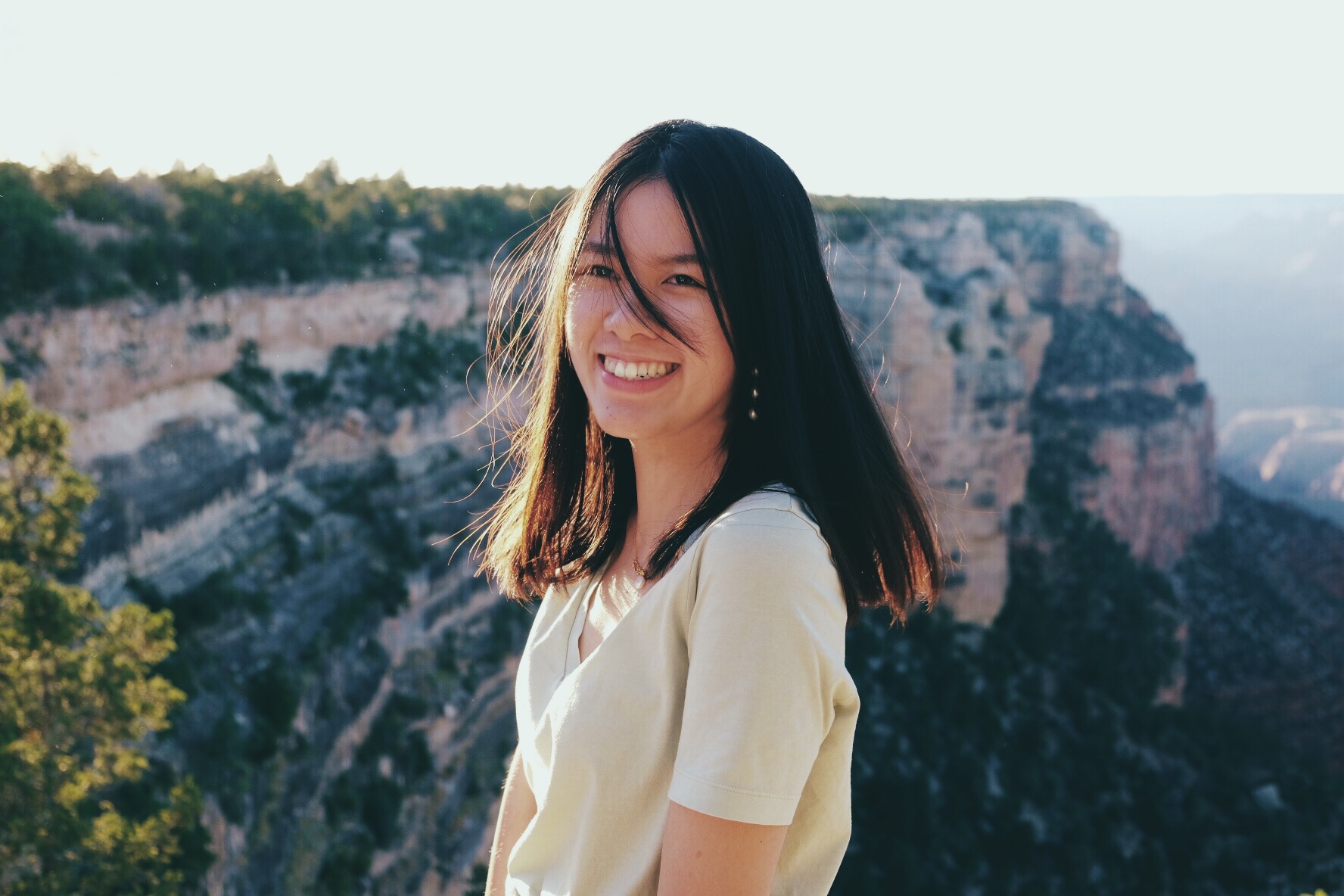
Hi, I am a 4th-year PhD student in Statistics at Columbia University, where I am very fortunate to work with Prof. John Cunningham. and Prof. David Blei. During my PhD, I’ve interned at Apple MLR hosted by Sinead Williamson. Previously, I received my master’s degree in Data Science at Columbia University, supervised by Prof. John Cunningham and Prof. Itsik Pe’er. I received my bachelor’s degree in Mathematics at Nanjing University.
Research Interests: In general I am interested in problems that invovle randomness and uncertainty, mostly through a probabilistic lens. Specifically, my recent focus has been:
- Probabilistic modeling: deep generative models (e.g. diffusion models, VAE, deep state space models), and Bayesian inference (e.g. variational inference, SMC)
- Uncertainty quantification: I’ve been mostly exploring the Bayesian approach, but I’m also open to other approaches
- Invariant prediction and out-of-distribution generalization